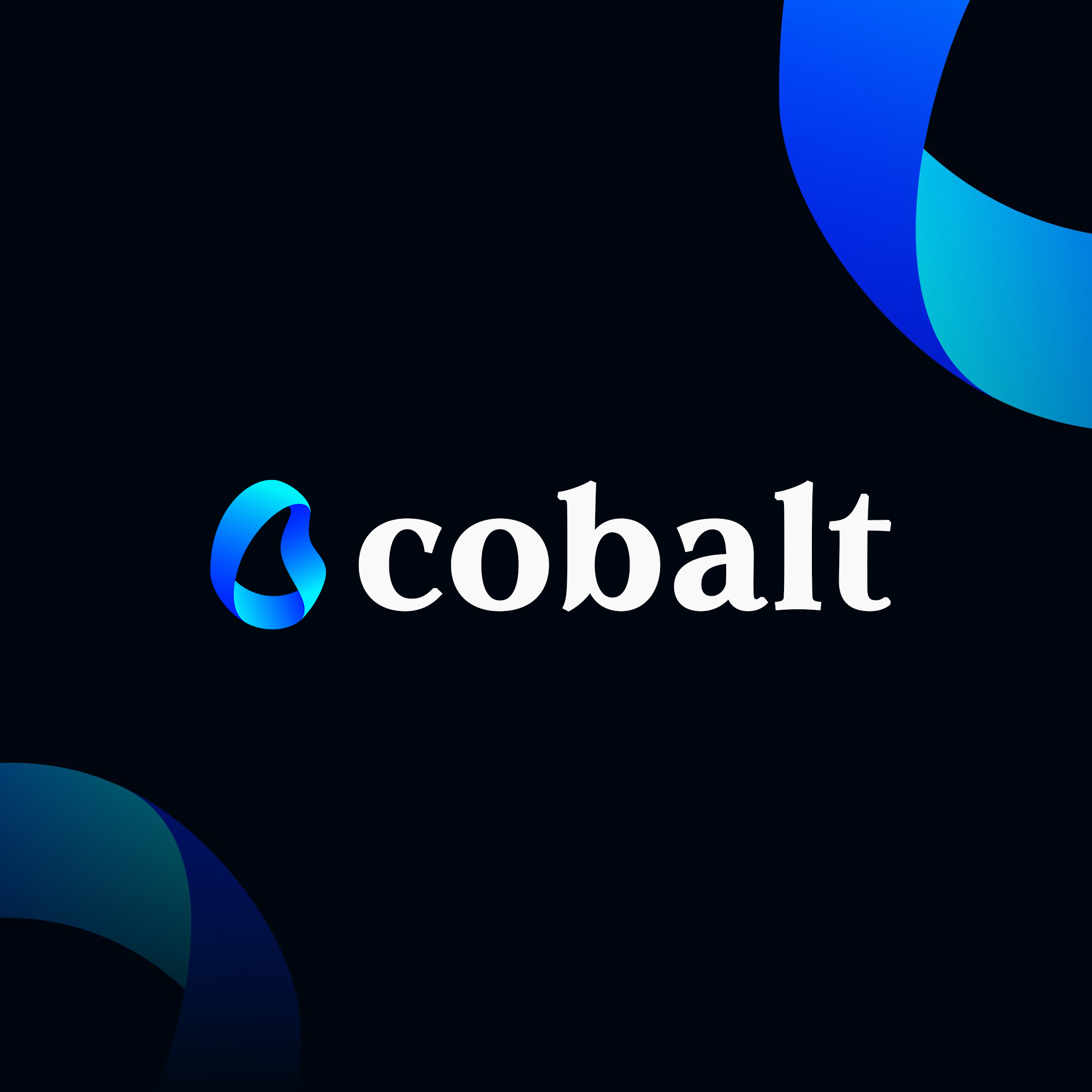
Why Your AI Needs Cobalt: Adapt, Diagnose, and Deploy with Confidence
Adapt AI to Your Use Case
The Challenge: Adapting State-of-the-Art Models to Industry-Specific Use Cases is Hard
Transforming a general-purpose model into an AI specialist for your industry is an ongoing process that continues throughout the lifecycle of the model. AI apps and agents are challenging to adapt because the interactions that occur are varied and constantly change with time. Consequently, enterprises are behind the curve in deploying LLM-based specialists and agents because they are not confident in their predictability and reliability (e.g. incorrect responses, hallucinations).
Our Solution: Cobalt – Mechanistic Interpretability for Model Evaluation
BluelightAI is a platform that helps users evaluate, adapt, and improve state of the art models for their use case. This platform provides a hub for comparing datasets, evaluation metrics, and models (open and proprietary) with specific, actionable insights into their capabilities and blind spots.
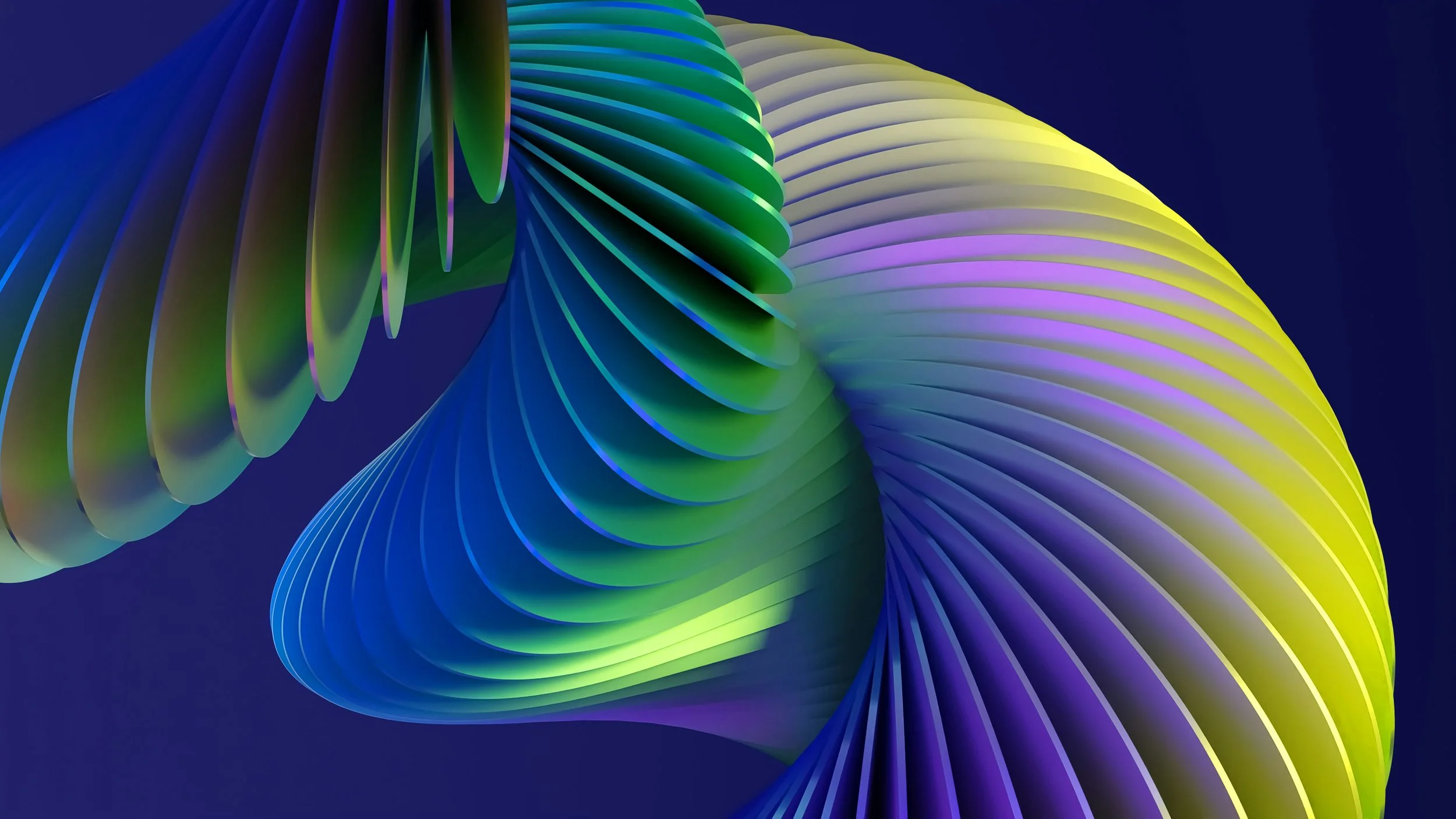
Mechanistic Interpretability in Practice: Applying TDA to Breast Cancer
This paper, which we shared last week, is a demonstration of how topological data analysis methods can be used for feature engineering and selection for data sets where there are many features, or columns in the data matrix.
In our Less Wrong post we pointed out that when a data set is “wide”, i.e. includes a large number of features, it is useful to compress the feature set into a graph structure where each node corresponds to a set of features. This gives an overview of what otherwise might be a feature set which is very hard to understand. Each data point can also be treated as a function on the nodes of the corresponding graph, so that one can examine and compare data points or collections of data points by “graph heat maps”, i.e. colorings of the nodes representing the functional values.
Mechanistic interpretability serves as the unifying macro concept here, as both sources leverage feature compression and graph-based visualization to transform high dimensional, opaque systems into interpretable structures that reveal underlying relationships. This paper studies a particular wide data set, where the features correspond to genes, with the entries being gene expression levels.
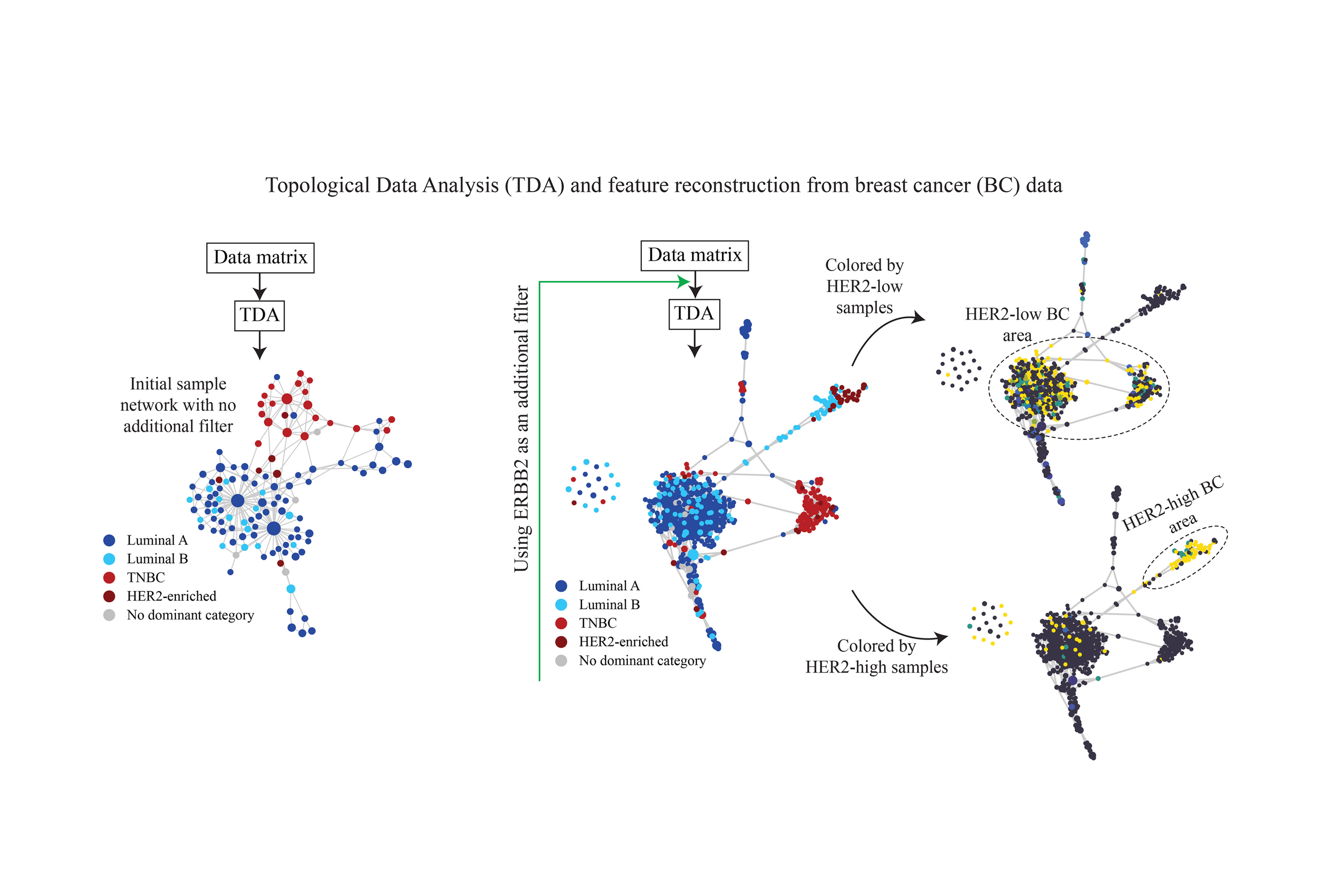
Topological Data Analysis Reveals a Subgroup of Luminal B Breast Cancer
This paper was originally published in the IEEE Open Journal of Engineering in Medicine and Biology.
Impact Statement:
High dimensional data is difficult to visualize or analyze. Reducing the dimension of data while preserving its veracity has become a key challenge for data science. A key tenet of data is its shape that can be topologically defined. In this study, we use the shape or topology of data to infer features that potentially carry valuable information. We use BC data as an example owing to its importance and complexity. The heterogeneity of BC data makes interpretation and prognosis difficult. We show that topological analysis of BC data and its graphical representation provide a natural subtyping in addition to parsing the associated features. Interestingly, the topological analysis points to a novel HER-2 positive luminal subtype, suggesting alternate therapeutic interventions.
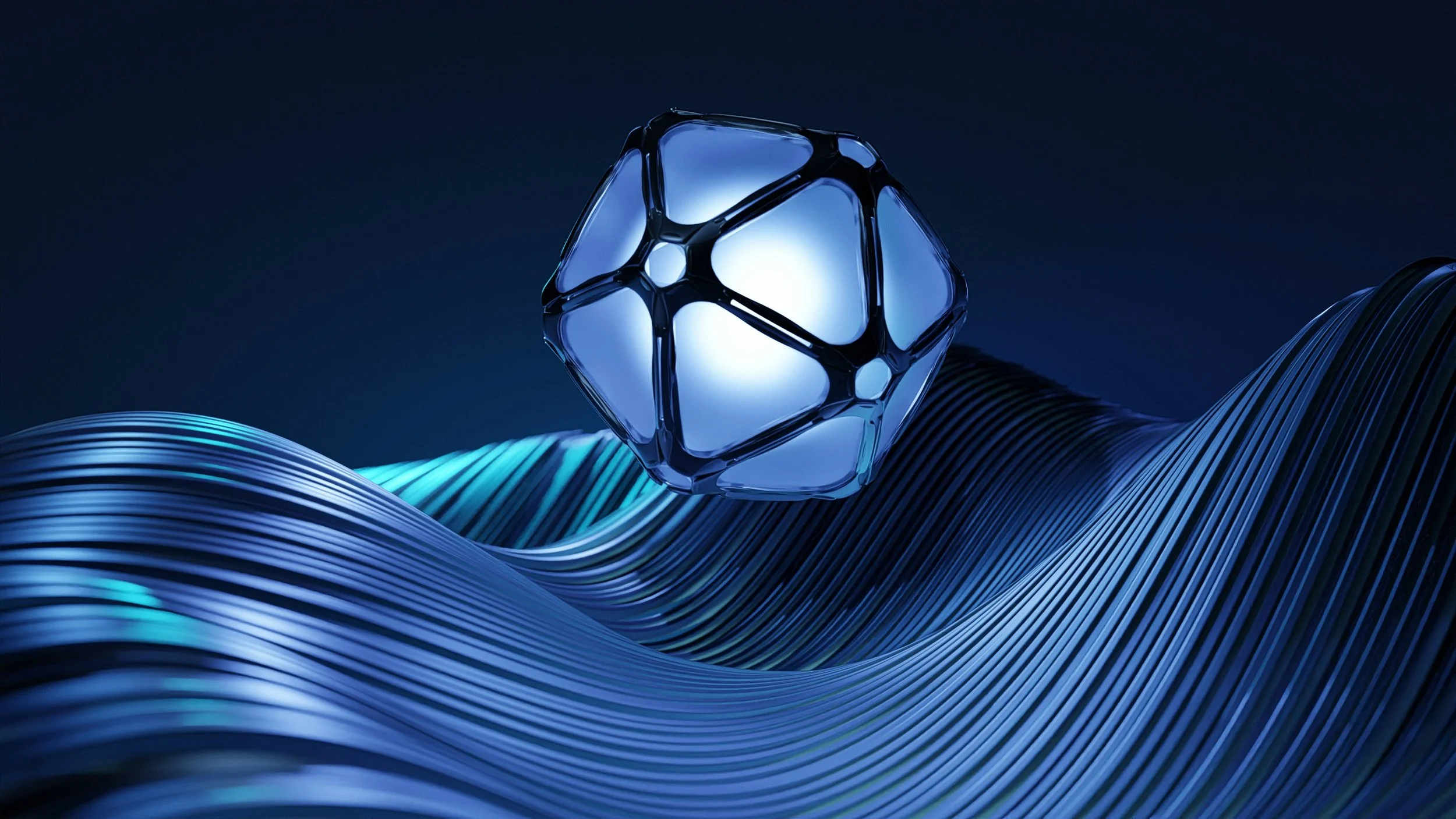
Next Generation AI Model Evaluation
Go beyond the leaderboard: How TDA uncovers what benchmark scores miss in model evaluation.
The evaluation of models is absolutely critical to the artificial intelligence enterprise. Without an array of evaluation methods, we will not be able to understand whether the models are doing what we want them to do, or what measures we should take to improve them. Another reason for the need for good evaluation measures is that once an AI model is deployed, we will find that the input data, the interaction of users with the model, and the user reactions to the output of the model will change over time. This means that not only do we need evaluation at the time of construction of the model, we will need to evaluate continually throughout the deployment lifecycle of the model.

New Release: Cobalt Version 0.3.9
Cobalt Version 0.3.9 is now available. You can install the latest version by running pip install --upgrade cobalt-ai
Features:
Group comparison in the UI now supports a choice of different statistical tests for numerical features.
In addition to the t-test, the Kolmogorov-Smirnov test and the Wilcoxon rank-sum test are supported, as well as a version of the t-test that uses permutation sampling to approximate the p-value instead of the t-distribution.
Workspace.get_group_neighbors() is a new method that finds a group af nearby neighbors of a given CobaltDataSubset. This neighborhood group can also be used as Group B in the group comparison UI.
The graph layout algorithm has been substantially improved and now presents cleaner, easier-to-read graphs. Some configuration options are available in cobalt.settings.
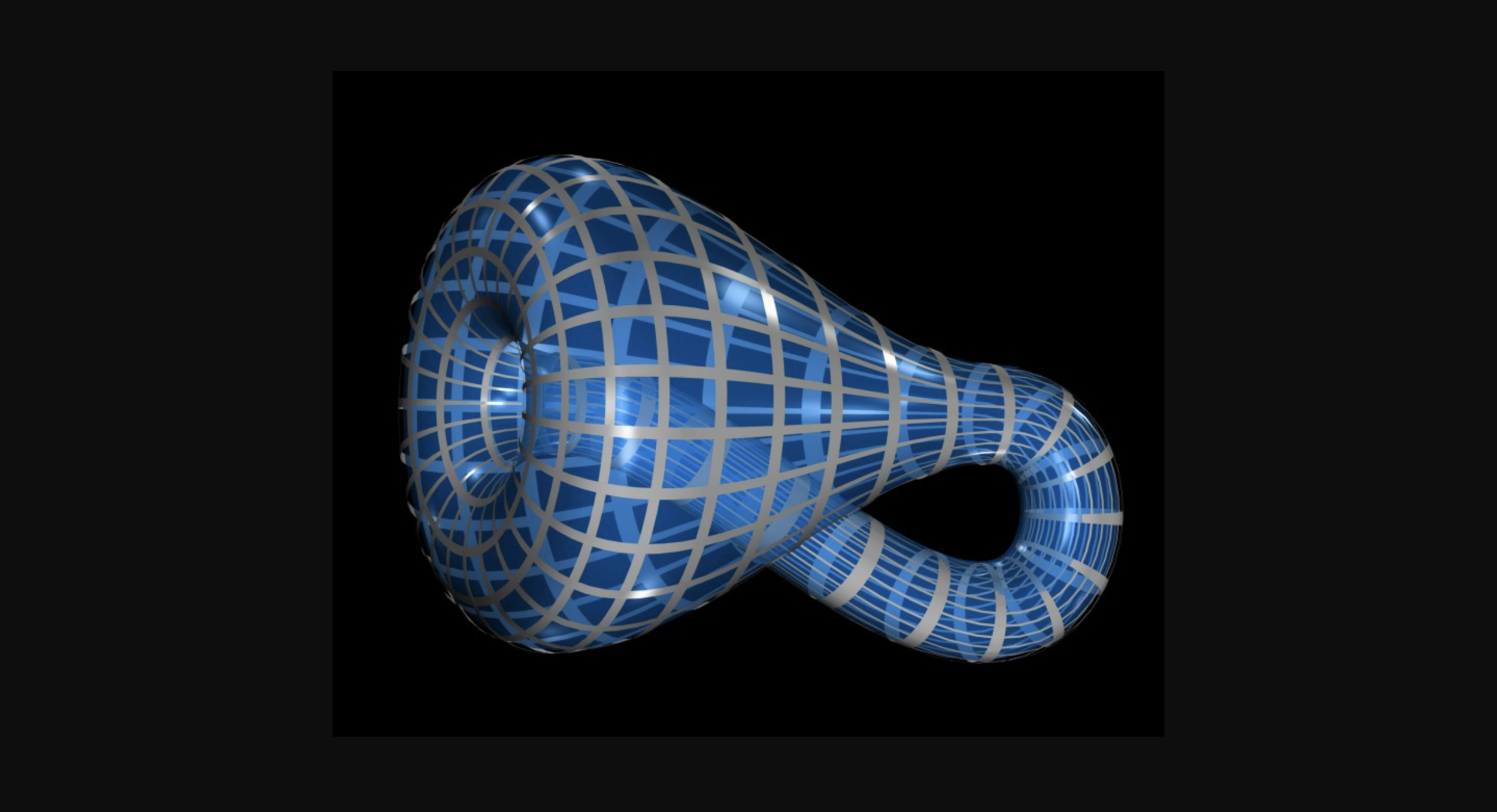
Improving CNNs with Klein Networks: A Topological Approach to AI
This article was originally published in LessWrong.
In our earlier post, we described how one could parametrize local image patches in natural images by a surface called a Klein bottle. In Love et al, we used this information to modify the convolutional neural network construction so as to incorporate information about the pixels in a small neighborhood of a given pixel in a systematic way. We found that we were able to improve performance in various ways. One obvious way is that the neural networks learned more quickly, and we therefore believe that they could learn on less data. Another very important point, though, was that the new networks were also able to generalize better. We carried out a synthetic experiment on MNIST, in which we introduced noise into MNIST. We then performed two experiments, one in which we trained on the original MNIST and evaluated the convolutional models on the “noisy” set, and another in which we trained on the noisy set and evaluated on the original set. The results are displayed below.
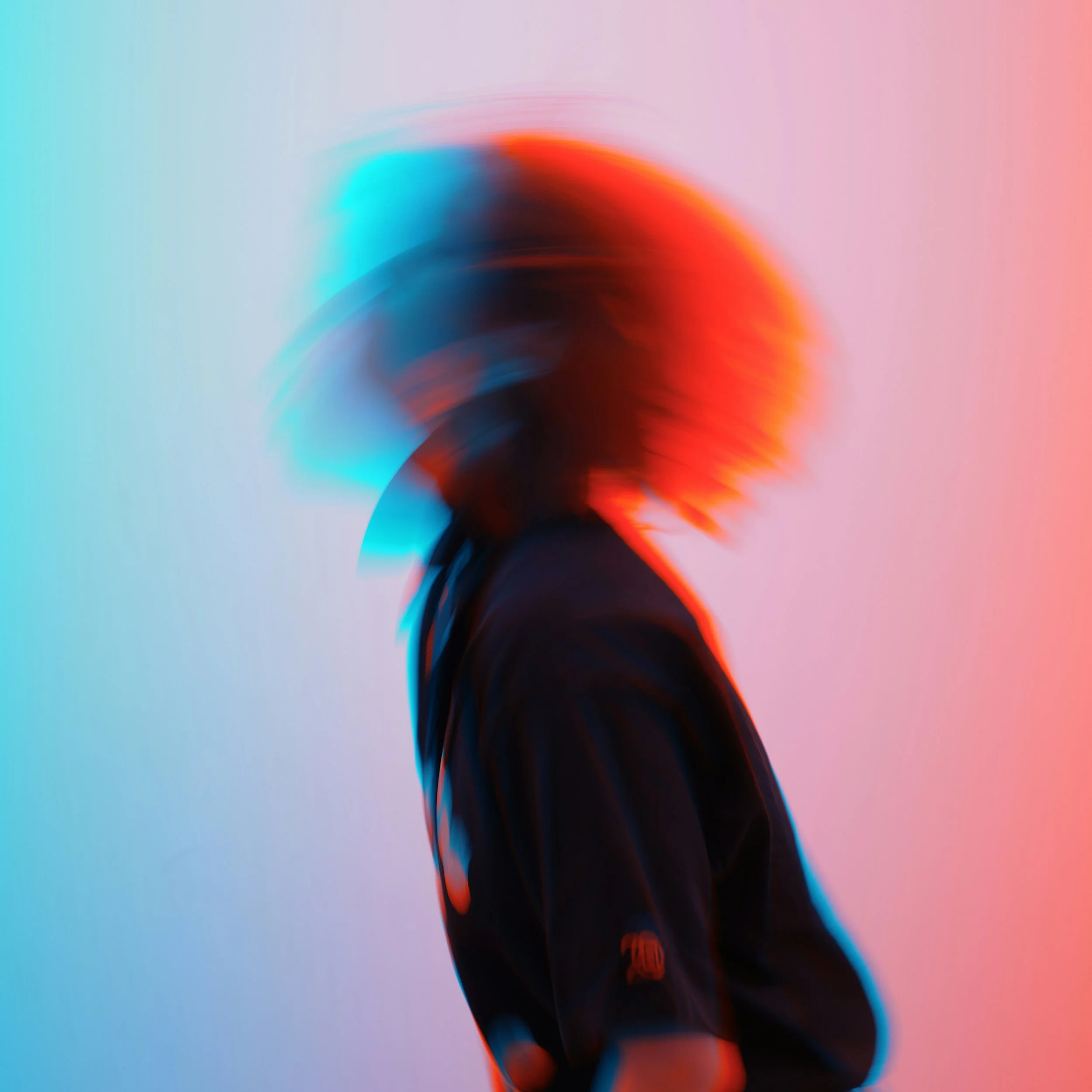
Evaluating LLM Hallucinations with BluelightAI Cobalt
Large language models are powerful tools for flexibly solving a wide variety of problems. But it’s surprisingly common for them to produce outputs that are untethered to reality. This phenomenon of hallucination is a major limiting factor for deploying LLMs in sensitive applications. If you want to deploy an LLM-based system in production, it’s important to understand the types of mistakes it may make, and use this knowledge to make decisions about what model to deploy and how to mitigate risks.
In this post, we’ll see how BluelightAI Cobalt can help understand a model’s tendency to hallucinate. In particular, we’ll identify certain types of inputs or questions where a model is more prone to make errors. Follow along in the Colab notebook!
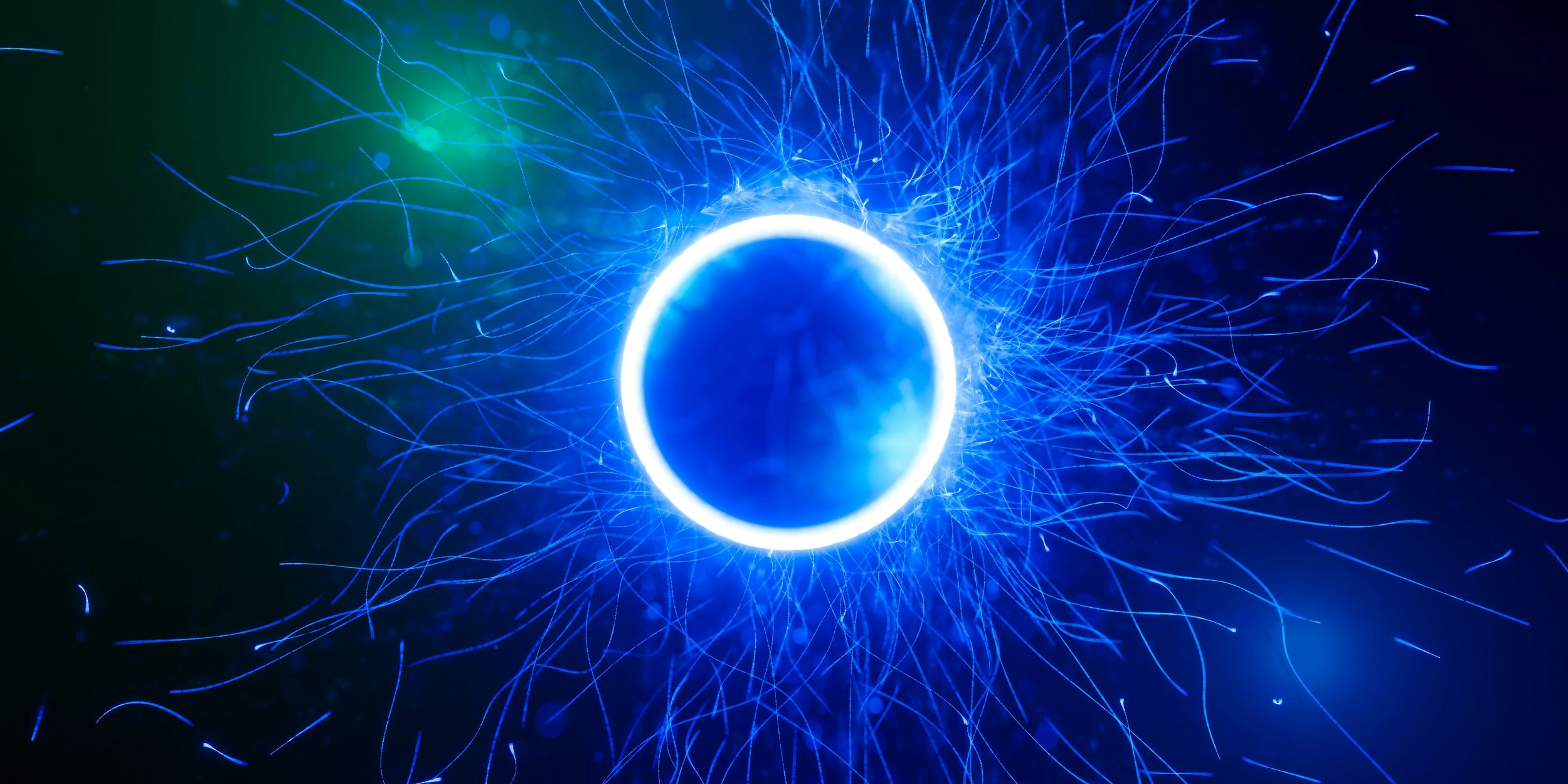
From Loops to Klein Bottles: Uncovering Hidden Topology in High Dimensional Data
Motivation: Dimensionality reduction is vital to the analysis of high dimensional data. It allows for better understanding of the data, so that one can formulate useful analyses. Dimensionality reduction that produces a set of points in a vector space of dimension n, where n s much smaller than the number of features N in the data set. If the number n is 1, 2, or 3, it is possible to visualize the data and obtain insights. If n is larger, then it is more difficult. One interesting situation, though, is where the data concentrates around a non-linear surface whose dimension is 1, 2, or 3, but can only be embedded in a dimension higher than 3. We will discuss such examples in this post.
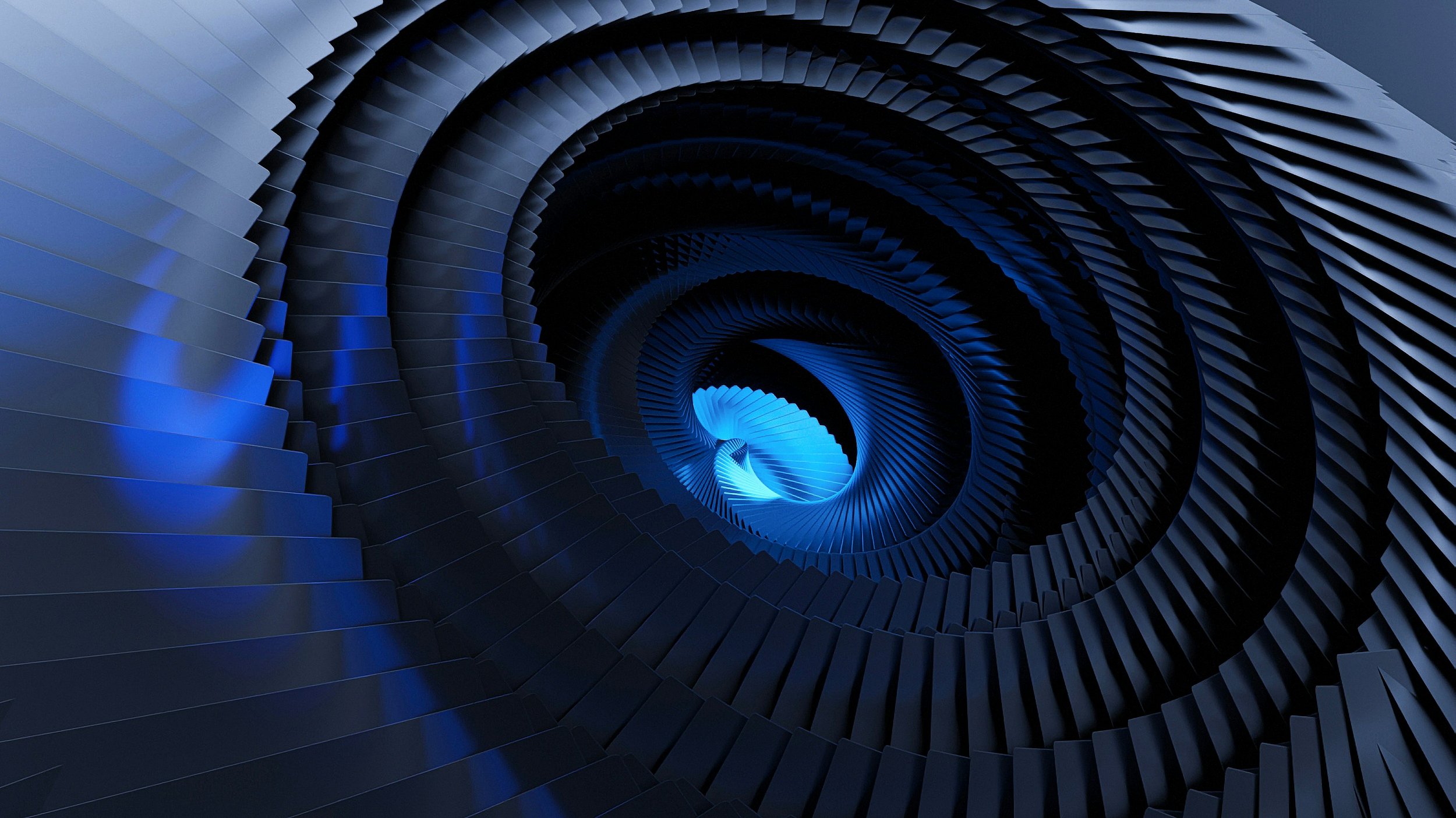
How to Use BluelightAI Cobalt with Tabular Data
BluelightAI Cobalt is built to quickly give you deep insights into complex data. You may have seen examples where Cobalt quickly reveals something hidden in text or image data, leveraging the power of neural embedding models. But what about tabular data, the often-underappreciated workhorse of machine learning and data science tasks? Can Cobalt bring the power of TDA to understanding structured tabular datasets?
Yes! Using tabular data in Cobalt is easy and straightforward. We’ll show how to do this with a quick exploration of a simple tabular dataset from the UCI repository. This dataset consists of physiochemical data on around 6500 samples of different wines, together with quality ratings and a tag for whether the wine is red or white.

Geometry of Features in Mechanistic Interpretability
This post is motivated by the observation in Open Problems in Mechanistic Interpretability by Sharkey, Chugtai, et al that “SDL (sparse dictionary learning) leaves feature geometry unexplained”, and that it is desirable to utilize geometric structures to gain interpretability for sparse autoencoder features.
We strongly agree, and the goal of this post is to describe one method for imposing such structures on data sets in general. Of course, it applies particularly to the case of sparse autoencoder features in LLM’s. The need for geometric structures on feature sets applies generally in the data science of wide data sets (those with many columns), such as occur as the activation data sets in complex neural networks. We will give some examples in the life sciences, and conclude with one derived from LLM’s.
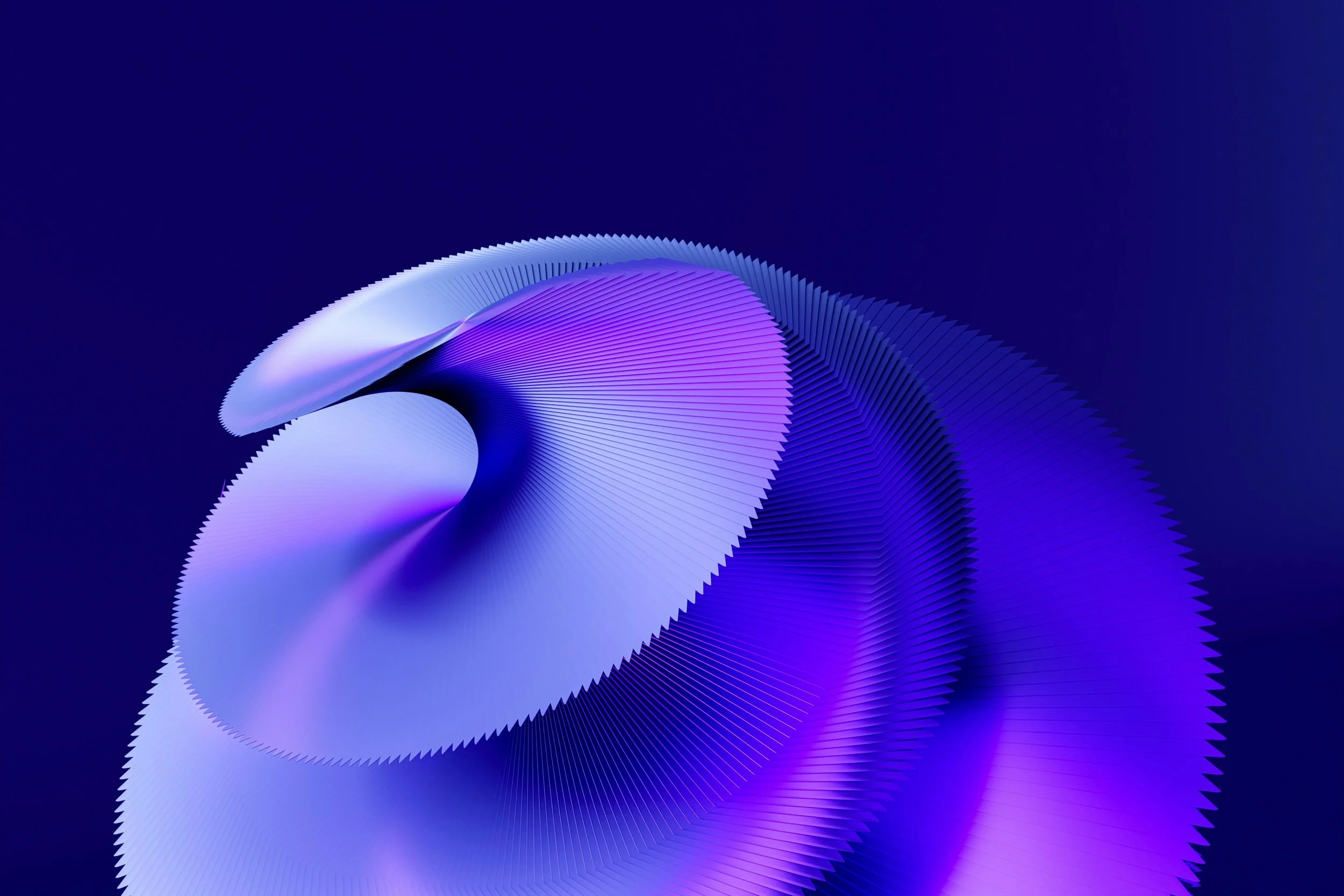
Topological Data Analysis and Mechanistic Interpretability
In this post, we’ll look at some ways to use topological data analysis (TDA) for mechanistic interpretability.
We’ll first show how one can apply TDA in a very simple way to the internals of convolutional neural networks to obtain information about the “responsibilities” of the various layers, as well as about the training process. For LLM’s, though, simply approaching weights or activations “raw” yields limited insights, and one needs additional methods like sparse autoencoders (SAEs) to obtain useful information about the internals. We will discuss this methodology, and give a few initial examples where TDA helps reveal structure in SAE feature geometry.